Exploration of Human-AI Cooperation in Neurorehabilitation Robotics
Progress in neurorehabilitation robotics has been significant, with a notable gap identified in Proximal Policy Optimization-based Reinforcement Learning (PbRL) research. This gap specifically pertains to cooperative multi-agent scenarios involving active human input. Addressing this void is crucial for advancing the understanding of human-AI dynamics in the context of neurorehabilitation. This article explores various aspects of this research, ranging from the introduction of the Human-Flexibility concept to the development of benchmarking suites and the adaptation of existing PbRL algorithms.
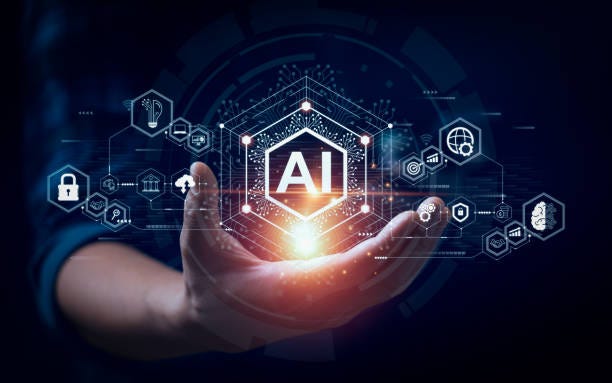
Key Contributions:
Introduction of Human-Flexibility Concept: Human-Flexibility adds depth to comprehend human-AI cooperation dynamics in PbRL. It emphasises the need to understand and accommodate the active input of humans in cooperative multi-agent scenarios, particularly in neurorehabilitation settings.
Benchmarking Suite Development: Creating specific domains requiring forced cooperation is a valuable tool for testing and comparing PbRL algorithms. These benchmarks simulate the challenges faced in cooperative multi-agent scenarios, contributing to a more nuanced evaluation of algorithmic performance.
Adapting Existing PbRL Algorithms: Leveraging existing PbRL algorithms for the two-agent setting demonstrates a practical approach. This adaptation facilitates a comparative analysis, shedding light on the strengths and limitations of established algorithms in scenarios involving active human input.
Clear Findings and Implications: The research highlights the challenges associated with human flexibility and limited policy access. These findings offer valuable insights for future research endeavours, guiding the development of more robust PbRL algorithms tailored for neurorehabilitation robotics.
Upper Bound Performance Identification: Specifying Specified Orchestration as an upper bound performance target is crucial for benchmarking progress. This identification establishes a clear goal for advancing PbRL algorithms in cooperative multi-agent scenarios.
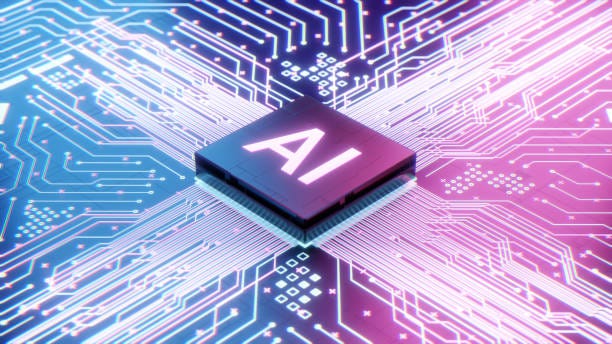
Areas for Further Discussion:
Exploration of Other PbRL Algorithms: Investigating alternative PbRL approaches explicitly designed for multi-agent settings may enhance performance, especially in highly human-flexible scenarios.
Human Feedback Complexity: Optimizing the design of preference queries to handle the complexity of human preferences in real-time interaction remains a key challenge. This area invites exploration for more efficient and user-friendly interaction.
Beyond Binary Preferences: The framework’s extension to incorporate richer forms of human feedback, such as graded preferences or subjective evaluations, warrants exploration. This expansion could further enhance the adaptability of PbRL algorithms.
Scalability and Real-World Application: Translating research findings into practical applications for human-robot collaboration in diverse environments remains a critical consideration. Exploring scalability and real-world applicability is essential for successfully implementing PbRL in neurorehabilitation robotics.
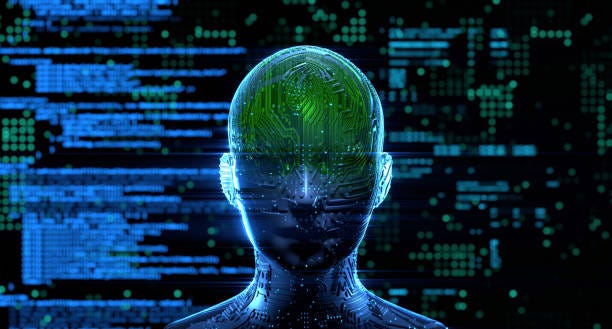
Application in Neurorehabilitation Robotics:
The application of PbRL in neurorehabilitation robotics holds immense promise and presents exciting possibilities for personalised therapy, adaptive assistance, motivational support, and shared decision-making.
Personalised Therapy through Preference Elicitation: Adapting the PbRL framework to robots assisting patients in rehabilitation exercises enables personalised therapy plans. The robot can tailor activities by understanding individual needs and preferences, leading to more effective and engaging therapy.
Adaptive Assistance and Feedback: Real-time learning of patient preferences allows the robot to adjust assistance levels dynamically. This adaptability ensures a more flexible and responsive therapy experience, aligning with the patient’s progress and fatigue levels over time.
Motivational Support and Task Selection: Beyond physical movements, the PbRL framework can inquire about preferred difficulty levels, therapy environments, and motivating feedback. This personalised approach enhances patient engagement, potentially improving adherence and overall outcomes.
Collaboration and Shared Decision-Making: The cooperative nature of PbRL fosters a sense of partnership between the patient and the robot. Actively involving patients in expressing preferences empowers them in their rehabilitation journey, promoting a collaborative and patient-centric approach.
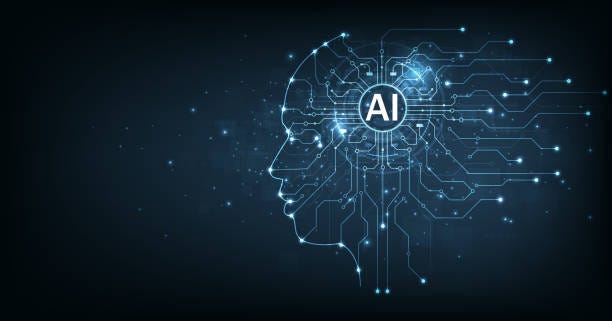
Challenges and Considerations:
Adapting PbRL Algorithms to Rehabilitation Tasks: Modifications to existing PbRL algorithms may be necessary to address the unique dynamics and uncertainties of neurorehabilitation settings effectively.
Ethical Considerations: Ensuring the PbRL framework respects patient autonomy and agency is crucial. Ethical considerations should prevent any perception of coercion or manipulation during rehabilitation.
Human-Robot Communication: Designing intuitive ways for patients to express preferences through the PbRL interface is essential for effective communication and building trust between humans and robots.
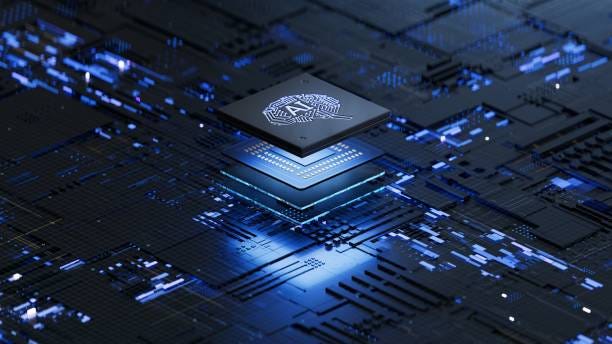
Despite these challenges, the potential benefits of PbRL in neurorehabilitation robotics are significant. Further research and development in this area is promising for revolutionising rehabilitation, making it more personalised, engaging, and ultimately more effective for patients. Exploring PbRL in cooperative multi-agent scenarios with active human input represents a crucial step towards unlocking the full potential of human-AI collaboration in neurorehabilitation robotics.
Zen KOH
Founder & Executive Director, MotusAcademy
Incoming President, IISART
Co-Founder & Global CEO, Fourier Intelligence
Zen is a visionary and pioneering influencer in the field of MedTech and Robotics for Rehabilitation. He has been involved in several successful start-ups and businesses in Singapore, Switzerland, and China for over two decades, providing medical devices, healthcare solutions, and services for people with disabilities and neurological patients. He was nominated as one of the 40 under 40 most influential industry leaders in MedTech in 2012.
Zen holds multiple leadership roles, including the incoming president and ambassador of the International Industry Society for Advanced Rehabilitation Technology (IISART), General Chair for RehabWeek 2023, co-founder and Executive Director of the Swiss-based MotusAcademy Association, Managing Editor of the Journal of Rehabilitation Methods and Technologies (JRMT), and co-founder and Global CEO of Fourier Intelligence Group. Under his leadership, Fourier has raised over USD100 million, notably in 2022, from renowned investors such as Saudi Aramco Prosperity 7 venture and Softbank Vision Fund 2.